To build a machine, one must know what its parts are and how they fit together. To understand the machine, one needs to know what each part does and how it contributes to its function. In other words, one should be able to explain the “mechanics” of how it works.
According to a philosophical approach called mechanism, humans are arguably a type of machine – and our ability to think, speak and understand the world is the result of a mechanical process we don’t understand.
To understand ourselves better, we can try to build machines that mimic our abilities. In doing so, we would have a mechanistic understanding of those machines. And the more of our behaviour the machine exhibits, the closer we might be to having a mechanistic explanation of our own minds.
This is what makes AI interesting from a philosophical point of view. Advanced models such as GPT4 and Midjourney can now mimic human conversation, pass professional exams and generate beautiful pictures with only a few words.
Yet, for all the progress, questions remain unanswered. How can we make something self-aware, or aware that others are aware? What is identity? What is meaning?
Although there are many competing philosophical descriptions of these things, they have all resisted mechanistic explanation.
In a sequence of papers accepted for the 16th Annual Conference in Artificial General Intelligence in Stockholm, I pose a mechanistic explanation for these phenomena. They explain how we may build a machine that’s aware of itself, of others, of itself as perceived by others, and so on.
Intelligence and intent
A lot of what we call intelligence boils down to making predictions about the world with incomplete information. The less information a machine needs to make accurate predictions, the more “intelligent” it is.
For any given task, there’s a limit to how much intelligence is actually useful. For example, most adults are smart enough to learn to drive a car, but more intelligence probably won’t make them a better driver.
My papers describe the upper limit of intelligence for a given task, and what is required to build a machine that attains it.
I named the idea Bennett’s Razor, which in non-technical terms is that “explanations should be no more specific than necessary”. This is distinct from the popular interpretation of Ockham’s Razor (and mathematical descriptions thereof), which is a preference for simpler explanations.
The difference is subtle, but significant. In an experiment comparing how much data AI systems need to learn simple maths, the AI that preferred less specific explanations outperformed one preferring simpler explanations by as much as 500%.
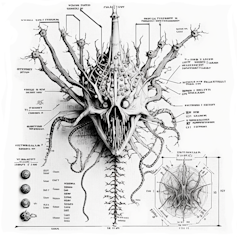
Exploring the implications of this discovery led me to a mechanistic explanation of meaning – something called “Gricean pragmatics”. This is a concept in philosophy of language that looks at how meaning is related to intent.
To survive, an animal needs to predict how its environment, including other animals, will act and react. You wouldn’t hesitate to leave a car unattended near a dog, but the same can’t be said of your rump steak lunch.
Being intelligent in a community means being able to infer the intent of others, which stems from their feelings and preferences. If a machine was to attain the upper limit of intelligence for a task that depends on interactions with a human, then it would also have to correctly infer intent.
And if a machine can ascribe intent to the events and experiences befalling it, this raises the question of identity and what it means to be aware of oneself and others.
Causality and identity
I see John wearing a raincoat when it rains. If I force John to wear a raincoat on a sunny day, will that bring rain?
Of course not! To a human, this is obvious. But the subtleties of cause and effect are more difficult to teach a machine (interested readers can check out The Book of Why by Judea Pearl and Dana Mackenzie).
To reason about these things, a machine needs to learn that “I caused it to happen” is different from “I saw it happen”. Typically, we’d program this understanding into it.
However, my work explains how we can build a machine that performs at the upper limit of intelligence for a task. Such a machine must, by definition, correctly identify cause and effect – and therefore also infer causal relations. My papers explore exactly how.
The implications of this are profound. If a machine learns “I caused it to happen”, then it must construct concepts of “I” (an identity for itself) and “it”.
The abilities to infer intent, to learn cause and effect, and to construct abstract identities are all linked. A machine that attains the upper limit of intelligence for a task must exhibit all these abilities.
This machine does not just construct an identity for itself, but for every aspect of every object that helps or hinders its ability to complete the task. It can then use its own preferences as a baseline to predict what others may do. This is similar to how humans tend to ascribe intent to non-human animals.
So what does it mean for AI?
Of course, the human mind is far more than the simple program used to conduct experiments in my research. My work provides a mathematical description of a possible causal pathway to creating a machine that is arguably self-aware. However, the specifics of engineering such a thing are far from solved.
For example, human-like intent would require human-like experiences and feelings, which is a difficult thing to engineer. Furthermore, we can’t easily test for the full richness of human consciousness. Consciousness is a broad and ambiguous concept that encompasses – but should be distinguished from – the more narrow claims above.
I have provided a mechanistic explanation of aspects of consciousness – but this alone does not capture the full richness of consciousness as humans experience it. This is only the beginning, and future research will need to expand on these arguments.
PhD Student, School of Computing, Australian National University